9.1 Introduction: Hypothesis Testing
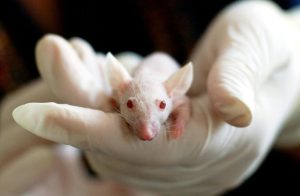
Learning Objectives
By the end of this chapter, the student should be able to:
- Identify the steps of hypothesis testing.
- Define null hypothesis, alternative hypothesis, test statistic, p-value, and statistical significance.
- Find and use a p-value to reach a decision about the null and alternative hypotheses and the underlying research question.
- State meaningful conclusions in the context of the problem at hand.
- Define Type I error and Type II error, and identify the type of error that researchers control.
One job of a statistician is to make statistical inferences about populations based on samples taken from the population. As we have seen, confidence intervals are one way to estimate a population parameter. Another way to make a statistical inference is to make a decision about a parameter based on a statistical hypothesis test. For example, a drug manufacturer claims their new drug is more effective at treating a disease compared to another treatment. A civil engineer wants to compare the average strength of a new concrete mix to the average strength of the traditional mix. An electrical engineer wants to determine if a new power supply has a lower failure rate compared to the current design. A statistician, scientist, or engineer may want to make a decision about or evaluate these types of claims. Hypothesis testing can be used to do this.
A hypothesis test involves collecting data from a sample and evaluating the data. Then a decision is made as to whether or not there is sufficient evidence, based upon analyses of the data, to claim a phenomena exists at all. The basic approach is to determine whether the observed data can or cannot be reasonably explained by a model that does not involve the phenomena at all. The process of hypothesis testing allows researchers to assess the validity of a claim or theory by following a structured approach. Here are some key reasons why hypothesis testing is used in research:
- Objectivity: Hypothesis testing provides a systematic and objective framework for evaluating the evidence. By following a formal procedure, scientists and engineers can reduce biases and subjectivity, ensuring that conclusions are based on data rather than personal beliefs or opinions.
- Decision Making: It helps scientists make informed decisions about whether to accept or reject a hypothesis. This is crucial in research and practical applications, such as determining the effectiveness of a new drug or the impact of a policy change.
- Quantifying Uncertainty: Hypothesis testing allows researchers to quantify the uncertainty associated with their conclusions. By calculating p-values and confidence intervals in conjunction with hypothesis tests, scientists can express the strength of the evidence and the likelihood of errors.
- Reproducibility: The structured nature of hypothesis testing makes research findings more reproducible. Other scientists can follow the same procedures to test the same hypotheses, which is fundamental for verifying results and building a cumulative body of scientific knowledge.
- Communication: Hypothesis testing provides a common language for scientists to communicate their findings. The concepts of null and alternative hypotheses, p-values, and statistical significance are widely understood and accepted in the scientific community, facilitating the sharing and interpretation of research results.
- Advancing Knowledge: Through the iterative process of hypothesis testing, scientists can refine theories and models. When hypotheses are tested and either rejected or not rejected, new insights are gained, leading to further questions and investigations that drive scientific progress. Hypothesis is often a first step and leads to new questions and additional testing.
Overall, hypothesis testing is a fundamental tool in scientific evidence-based research. In this chapter, you will conduct hypothesis tests on means and proportions. You will also learn about the errors associated with these tests.